Meet our experts: Graham Cook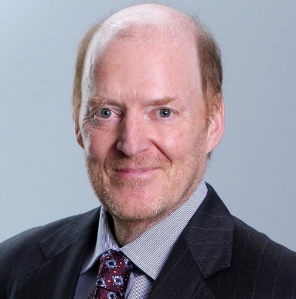
Dr. Graham Cook, Principal, Insurance Consultant, who is on Floodlight’s advisory team is the chief architect of the Floodlight CAT model. Dr. Cook is an industry veteran with over three decades of experience and practice in this field. He has published widely and has consulted to various re/insurance companies, various agencies, and startups on catastrophic risk in North America, Asia, and Europe. He has a PhD in Civil/Structural engineering from Johns Hopkins University. He is a member of the Engineers Australia, Working Group on Disaster Risk Reduction and Responses.
Graham is an experienced insurance and reinsurance professional. He is responsible for leading teams researching, developing and evaluating third party and in house catastrophe and financial models. He has lectured on catastrophe models and ESG for re/insurance companies in the US, Europe, Asia and Australia.
Graham works in the area of catastrophic (Cat) insurance models and climate risk models. Graham notes that Cat models utilize geospatial data and analysis in several key ways to assess and predict the potential impact of natural disasters on insured properties. Geospatial data collected from actual events is used to calibrate and validate cat models, ensuring that the models accurately reflect real-world conditions and outcomes. Traditionally, cat models are used to evaluate the financial impacts of natural disasters like hurricanes, earthquakes, and floods. They focus on the immediate risks posed by these events to insurance and reinsurance portfolios, estimating potential losses based on historical data and hazard analysis.
Can you elaborate on best practices in the context of cat models?
To effectively manage catastrophic risks, it is essential to leverage the strengths of advanced insurance models. This begins with auditing and enhancing data quality, which ensures that the foundation of any model is accurate and reliable. Understanding the science behind these models, including the nuances of model changes, is crucial for interpreting results and making informed adjustments.
By comparing outputs from multiple models, we can identify inconsistencies and validate the reliability of predictions. Sensitivity analyses further refine these results, helping us understand how different variables impact outcomes. Blending these insights with expert judgments allows for a more comprehensive and nuanced approach to risk management. Adjusting models for non-modeled perils, such as unexpected or rare events, ensures that all potential risks are accounted for. Employing diverse metrics like Value at Risk (VaR) and Tail Value at Risk (TVaR) provides a robust framework for assessing these risks. These are relevant in climate risk models at the asset level. A commercial REIT or solar or wind farm’s risk from multiple hazards can be estimated using similar procedure.
Simple deterministic methods offer additional insights, particularly in identifying exposure concentrations that could amplify losses. Finally, integrating underwriting and actuarial judgments into the process ensures that these models are not just theoretically sound but also practically applicable, making them powerful tools in the field of catastrophic insurance.
How do cat models vary from climate models?
Catastrophe models and climate models serve different purposes and have distinct limitations. Catastrophe models, while not designed as climate models, do implicitly account for moderate increases in losses attributed to climate change. However, they are focused on accurately modeling extreme weather events at a high spatial resolution (10m to 10km), making them more suitable for pricing risks in the insurance industry, which operates on an annual basis.
In contrast, climate models, which divide the world into larger cells (ranging from a kilometer to hundreds of kilometers), struggle to accurately simulate the specifics of hurricanes—such as intensity, frequency, and landfall locations. Additionally, climate models are not practical for pricing risks due to their inability to precisely model extreme weather events and the high cost of running them over the necessary time and spatial dimensions. Moreover, since climate change occurs over extended periods, its impacts do not align directly with the annual pricing structure of insurance policies.